Foundation Model Approach to Automatically Discover Geofeatures
Foundation Model Approach to Automatically Discover Geofeatures
Inspired by recent computer vision breakthroughs in machine-learning (AI/ML) we are developing a seismic foundation model (FM) based approach to assist in automatically discovering geological features of interest across diverse geological environments. Whether for a single survey or hundreds of contiguous region-wide surveys, our approach permits subject matter experts to rapidly explore major geological features automatically.
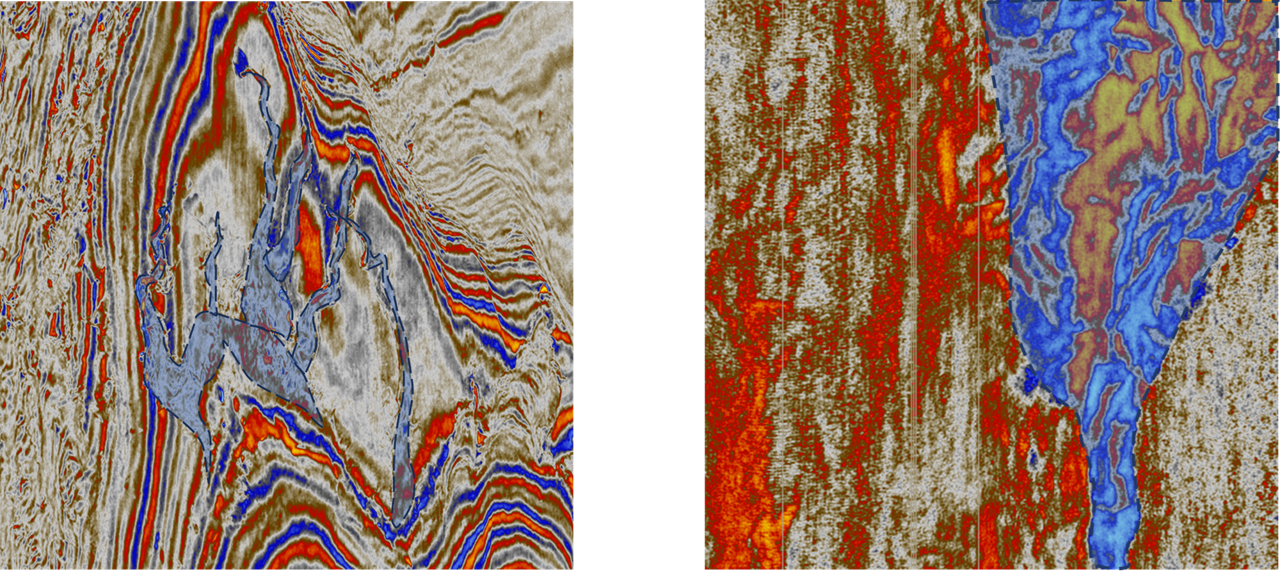
Figure-1: Channels (left) and Fans (right) depicted above with blue shading. Fans are typically large-scale structures, and their automatic detection is a challenging task to accomplish using traditional ML approaches. Our FM-based approach can discover such geofeatures with varying length scales.
The system employs a large seismic FM to learn multi-scale features in seismic data, and during inference this semantically structured feature space is employed to discover structural, stratigraphic and depositional geofeatures. Since this FM feature space is semantically structured it can comprehend geological nuances and allows for wide variations in geological attributes. This enables the expert to rapidly navigate a region to explore features discovered and to narrow down to a region-of-interest for more in-depth analysis. Some examples of its current capabilities are highlighted in Figures 1, 2 and 4. Figure 3 provides the reader with a thumbnail sketch of the system architecture. We are working towards integrating functionality offered by such seismic FMs in Delfi next generation subsurface interpretation workflows.
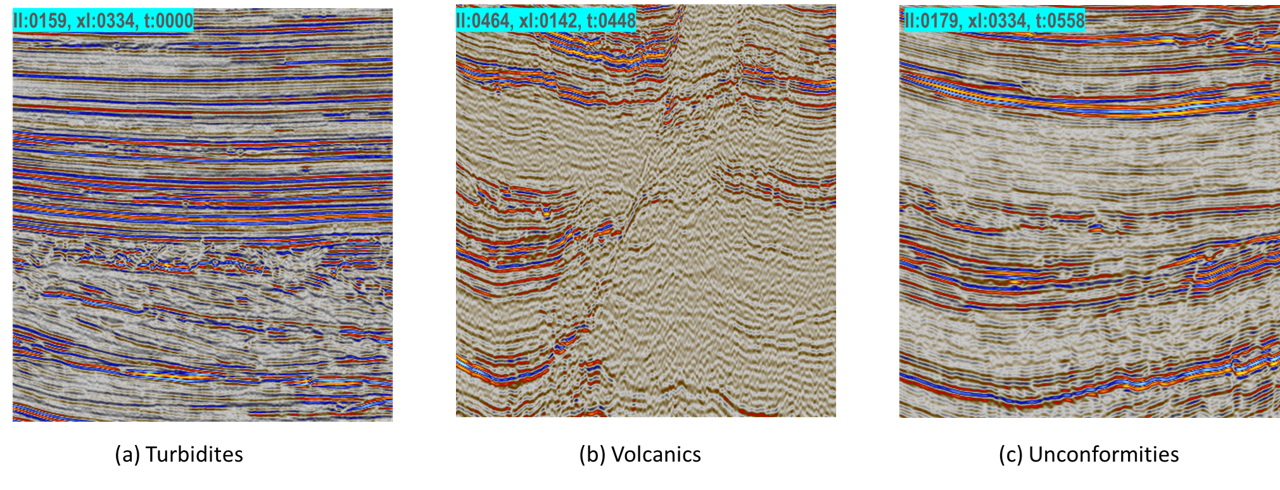
Figure-2: Examples of the structural, stratigraphic and depositional features within Parihaka as discovered by our seismic foundation model (FM) based approach. The FM was not trained using data from Parihaka. From left to right: Turbidites, Volcanics, and Unconformities. The FM-based system will automatically discover and flag such geofeatures for further review by the expert.
The figures display a few geofeatures as discovered from Parihaka which lies to the Northwestern part of offshore Taranaki Basin, New Zealand. The seismic FM was not trained using data from Parihaka. The basin has a sedimentation history extending from the Late Cretaceous period to the present. Tectonic activity within the basin is in a continuation phase since its initiation and still there are many active fault systems within the basin. The basin possesses a complex faulted style architecture (Kumar et al., 2016).
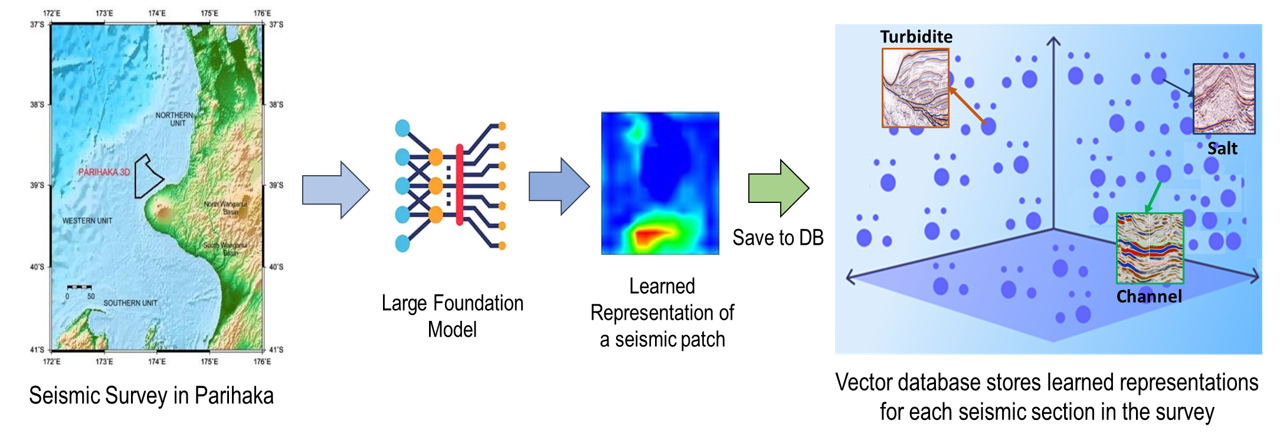
Figure-3: (Left) Regional view of the New Zealand with many surveys. (Mid) The seismic FM will scan sections of a new survey at a rate of a second for salient geofeatures. (Right) A distributed vector database will index geofeatures discovered in individual surveys. Since such vector databases can horizontally scale, the expert can revisit salient geofeatures across 100s of surveys in a matter of seconds.
Acknowledgements: We thank the New Zealand Petroleum and Minerals (NZPM), Ministry of Business, Innovation & Employment, New Zealand, for providing the access to data used in our study.
References
Kumar, Priyadarshi. (2016). Application of geometric attributes for interpreting faults from seismic data: An example from Taranaki Basin, New Zealand. 2077-2081. 10.1190/segam2016-13944184.1.
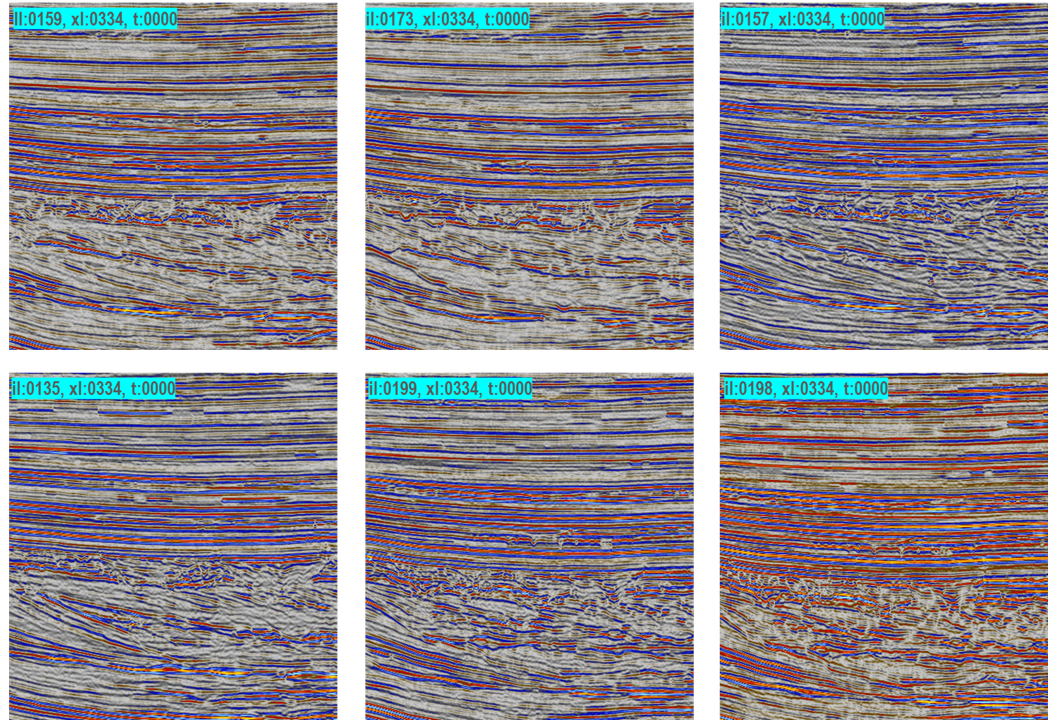
Figure-4: A closer look at a few turbidites sections discovered for Parihaka. The varied local characteristics suggest that our FM-based approach accounts for the semantic nuances of geofeatures.