At the forefront of new ideas across the energy sector
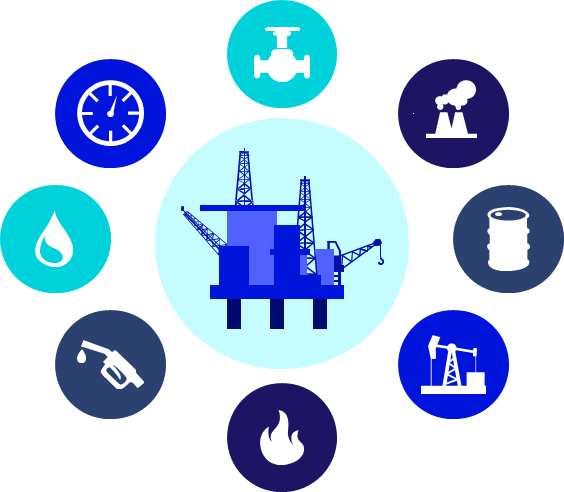
Trusting GenAI: Crafting a Robust Platform Strategy for the Energy Sector
We stand on the brink of a profound transformation. Artificial intelligence is rapidly reshaping the entire value chain within the energy sector, with the emergence of Generative AI (GenAI) compressing years of evolution into mere months. However, a critical challenge persists: fostering trust in AI-driven decisions, especially in an industry where the stakes are exceptionally high.
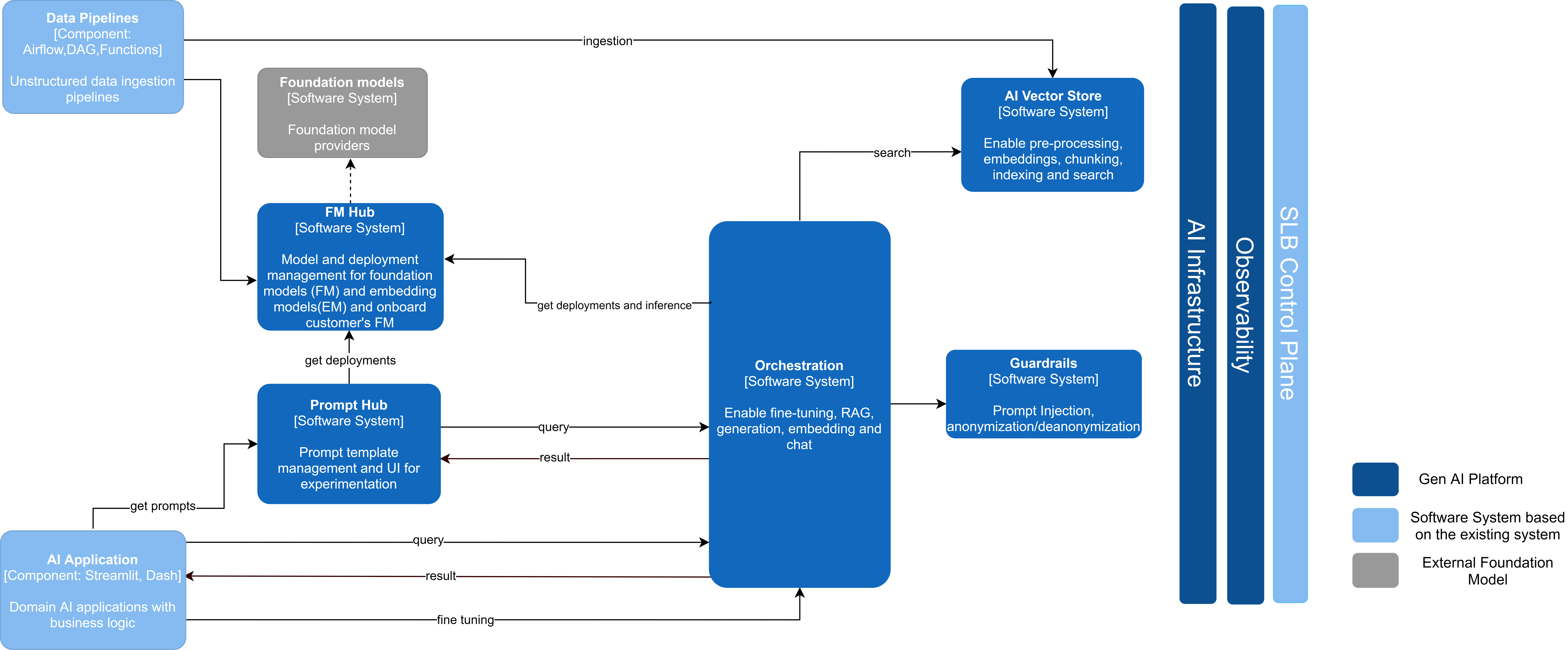
A Generative AI Platform: Powering the Future of the Energy Industry
Revolutionizing Operations with Advanced AI Integration.
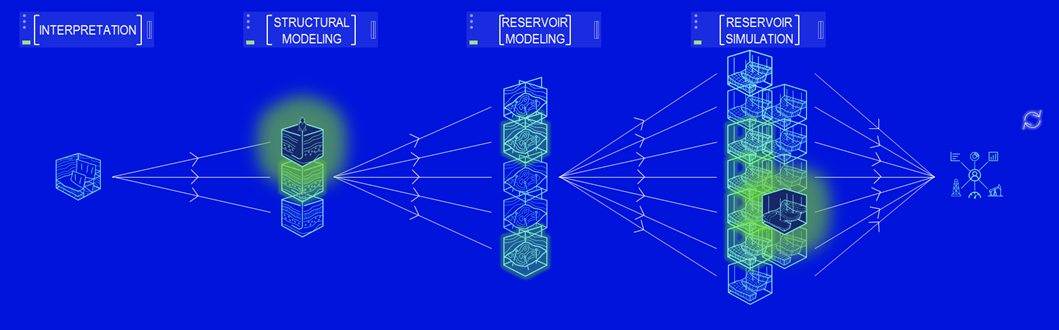
Driving Innovation in Subsurface Simulation Workflow Using Generative Artificial Intelligence
Artificial intelligence (AI) is transforming subsurface energy recovery by enhancing the accuracy and efficiency of exploration, drilling, reservoir and production processes. AI algorithms analyze vast amounts of geological and geophysical data to identify optimal drilling locations, predict reservoir behavior, and optimize production strategies, leading to more efficient resource extraction and reduced operational costs.
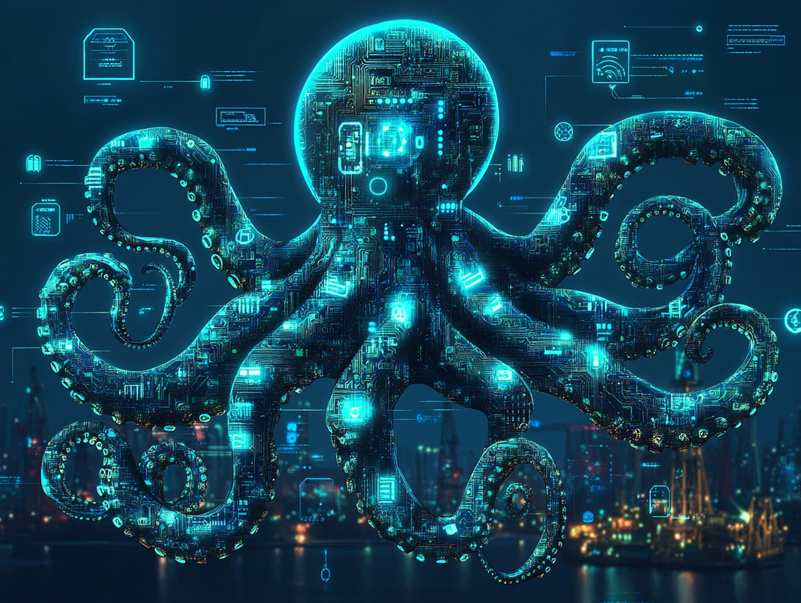
Agentic Workflows: Powering Intelligent Energy Solutions
Harnessing AI agents for complex energy challenges
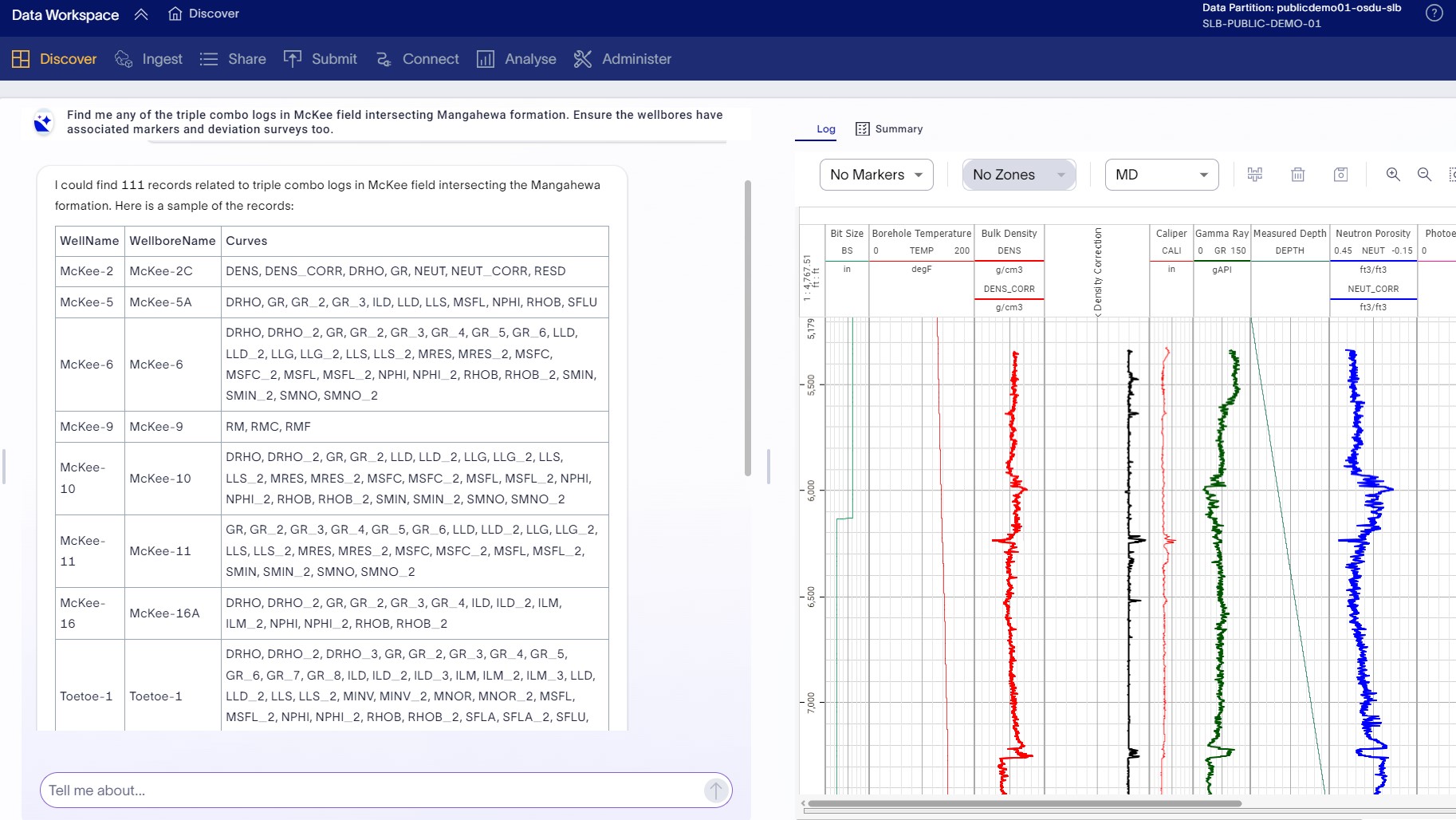
Data Workspace Conversational insights: Revolutionizing Data Discovery and Insight extraction
Over the years, oil exploration and production companies have amassed enormous amounts of data spread across various modalities ranging from structured records stored in relational databases to unstructured data such as well documents, seismic surveys, well logs, and maps. As the volume of data grows, navigating and deriving valuable insights from it becomes increasingly challenging. Traditional search techniques often struggle to manage the complexity and variety of these datasets, resulting in inefficiencies and missed opportunities for informed decision-making. Built upon the capabilities of Generative AI, Data Workspace (DW) conversational insights empower domain scientists and technologists to effortlessly access, extract, and assimilate information from these diverse sources to accelerate process of accurate data interpretation, enhance existing data repositories, and drive informed strategic decisions.
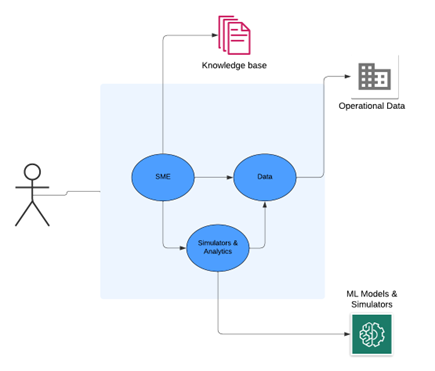
Smart Production Assistant: Transforming Industrial Operations with Generative AI
We’ve all seen how large language models (LLMs) can fetch details from documents and answer questions. But what if we took things further? Imagine an LLM that not only pulls information from real-time operational data but also blends it with industry knowledge from manuals, design documents, and best practices. Picture it is using standard oil and gas simulators like PIPESIM or SYMMETRY, and even running advanced machine learning models on the fly to tackle complex problems. That’s where true innovation lies—combining live data, industry expertise, simulators, and AI-driven tools to address challenges with unmatched efficiency.
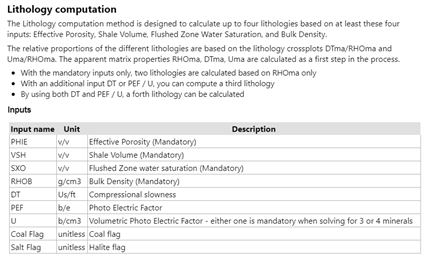
Accelerating Wellbore Domain Interpretations Using Generative AI and Knowledge Graphs
The petroleum industry relies heavily on accurate wellbore domain interpretation for effective resource management and extraction. Traditionally, this process involves numerous complex and time-consuming steps, such as data normalization, unit harmonization, and depth matching, often requiring extensive expertise. This paper presents an AI-powered solution designed to automate the creation of wellbore interpretation workflows, significantly enhancing efficiency and accuracy.
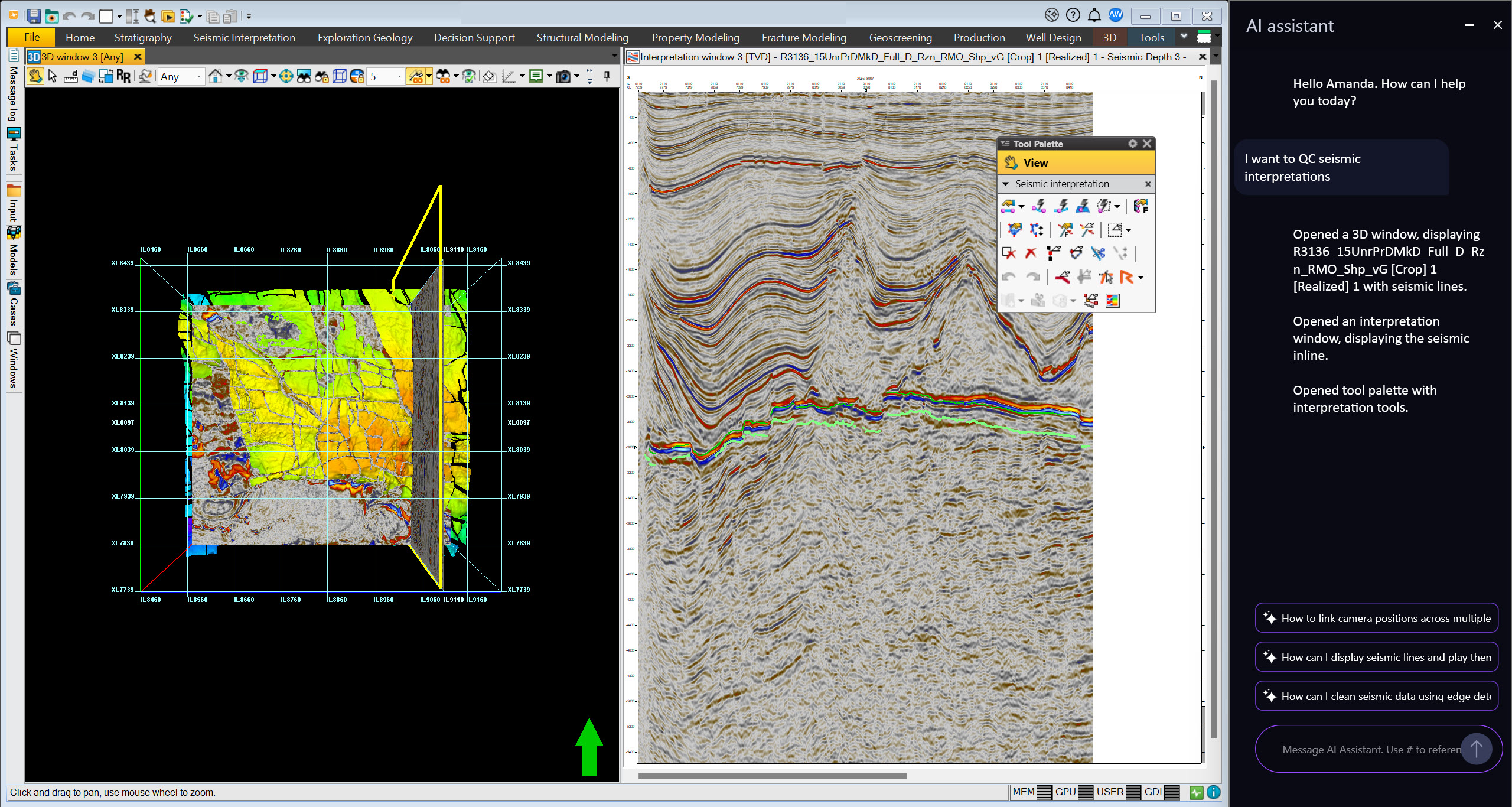
Generative AI for Subsurface Workflows in Petrel
Petrel™ subsurface software is a powerful subsurface tool designed to tackle complex problems. However, both beginner and advanced users often spend significant time not on scientific tasks, but on complex dialogs, visualizations, and displays. These tasks include adjusting labels, color tables, and configuring settings across multiple windows. While these activities are important for creating a final product, they can detract from the larger workflow at hand.
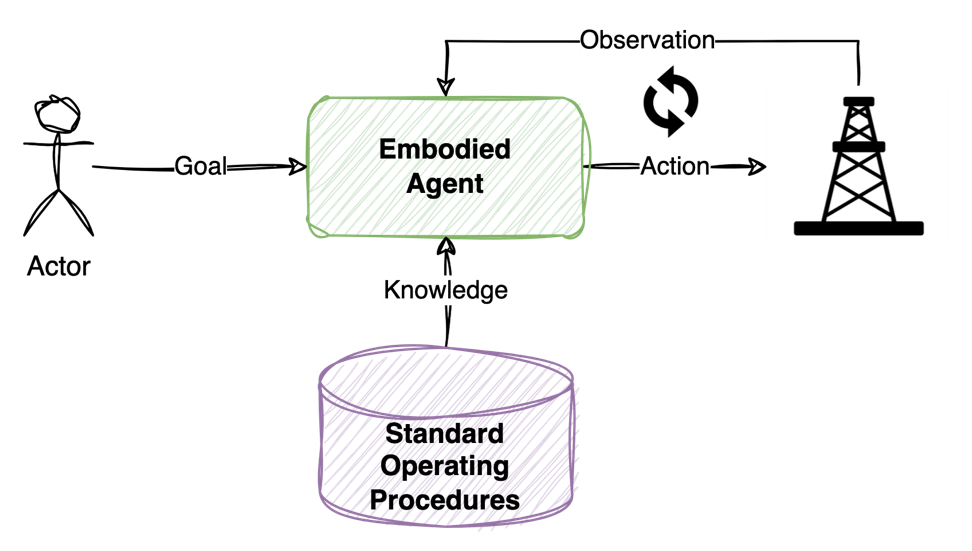
GenAI Agents in Drilling Process Orchestration
Automation of Wellsite operations is not new, over the last 10 years the industry has seen several innovations in this area both in Drilling and Wireline operations. For example DrillOps has been offering drilling automation and advisory capabilities for some time now, similarly the Neuro Autonomous Well Intervention has enabled automation of Wireline and Coiled Tubing intervention operations. The benefits of automation are not always crew size reduction in many cases we have seen improvements in performance, better adherence to procedures and improved consistency across operations. A human is always in the loop and in many cases the automation systems have to work together with humans to achieve a goal.
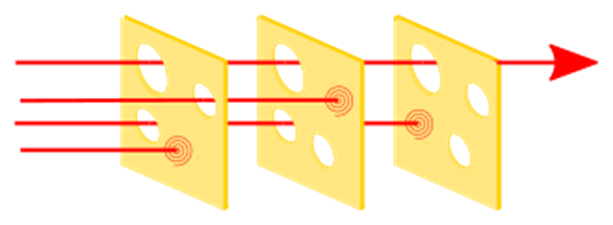
Augmenting Drilling Risk Management with Generative AI
Oilfield operators and oilfield service providers currently manage well construction risks by analysing historical daily drilling reports (DDRs) to identify significant events, their relevance to current operations, and appropriate risk control measures. However, these reports often lack proper details, and near misses are frequently underreported, leading to a higher risk of high-impact events that are challenging to predict and mitigate. This post discusses how an on-premises implementation of generative AI workflows enhances the identification, classification, characterization, and summarization of past drilling events from historical DDR activity descriptions.
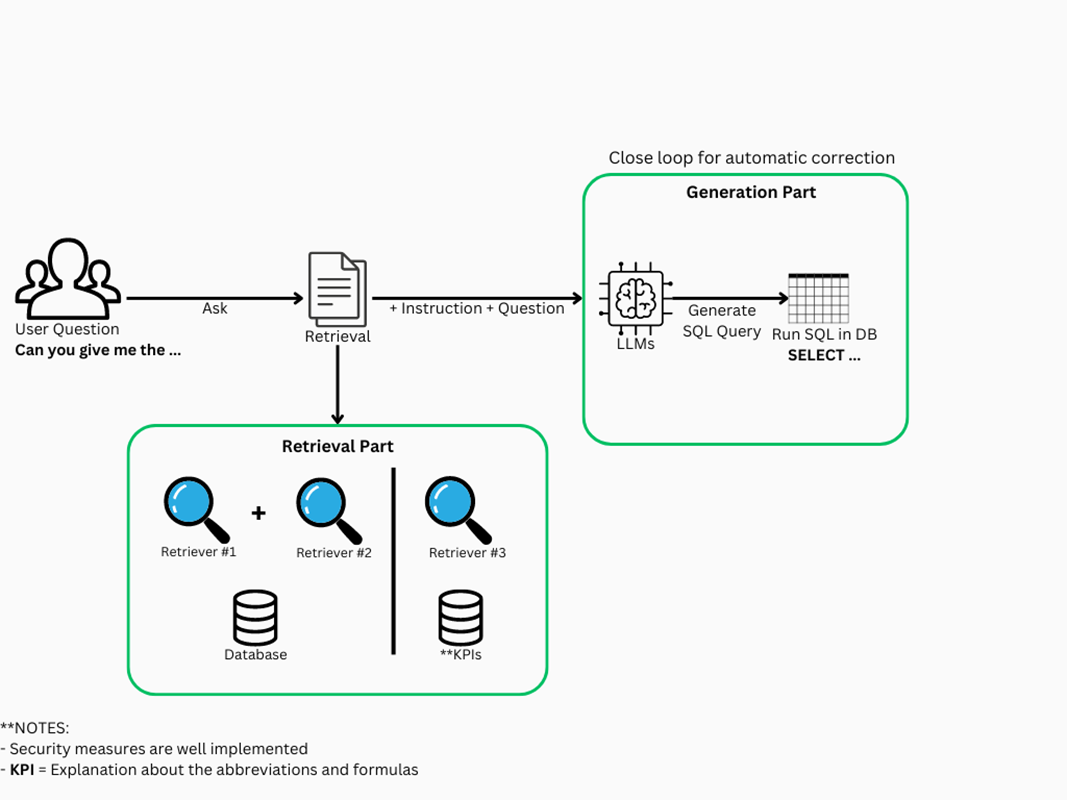
Empowering Drilling and Optimization with Generative AI
Over the last 10 years, no industry has faced more pressure and scrutiny to gain efficiency than the oil and gas (O&G) industry. Drilling engineers are tasked with leveraging data to optimize design and operations. O&G companies have collected and stored large amounts of high-frequency sensor data and reporting data. Efficient data access is fundamental for upstream projects, and data operations skills are critical for drilling engineers. However, data operations have not become a core competency for most of the drilling engineering community.
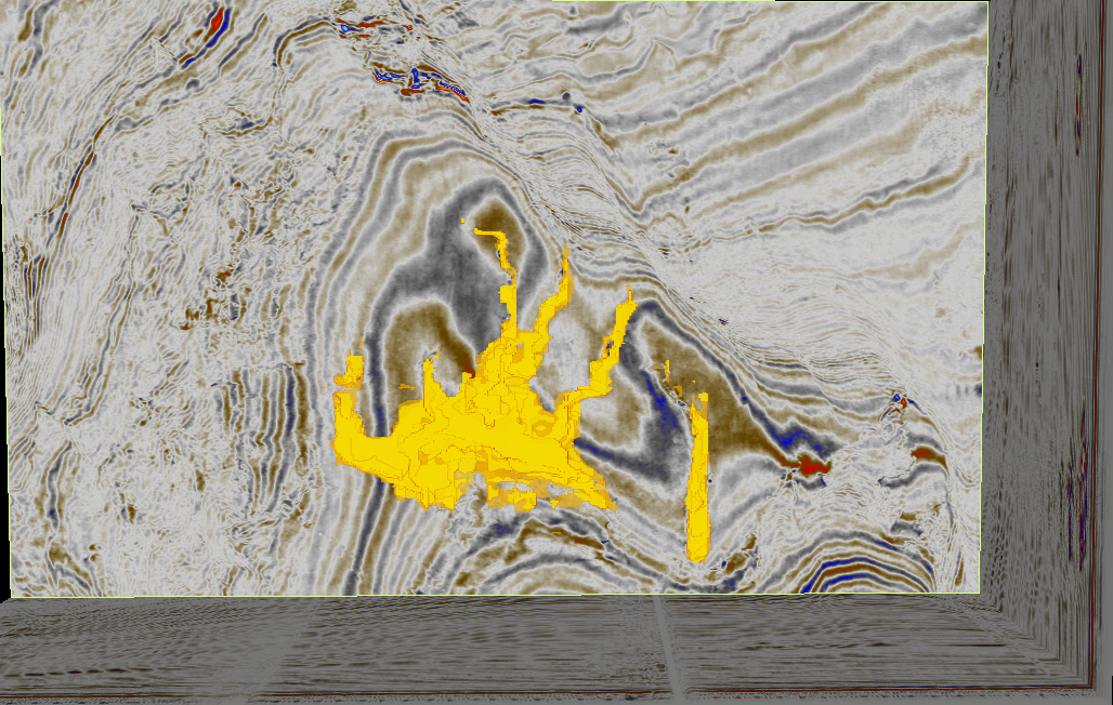
Pretrained Models for Rapid Geofeature Segmentation
Transformer-based architectures can scale complex models and meaningfully process large volumes of seismic data. Architectural constructs within these models can allow multi-scale capture of seismic data. Self-supervised learning methods allow these models to be trained on very large datasets without needing labeled data. Models built in such a fashion, so-called “pretrained models”, can exhibit feature representations which are more complete, provide better generalization, and implicitly capture diverse domain-specific semantic nuances.
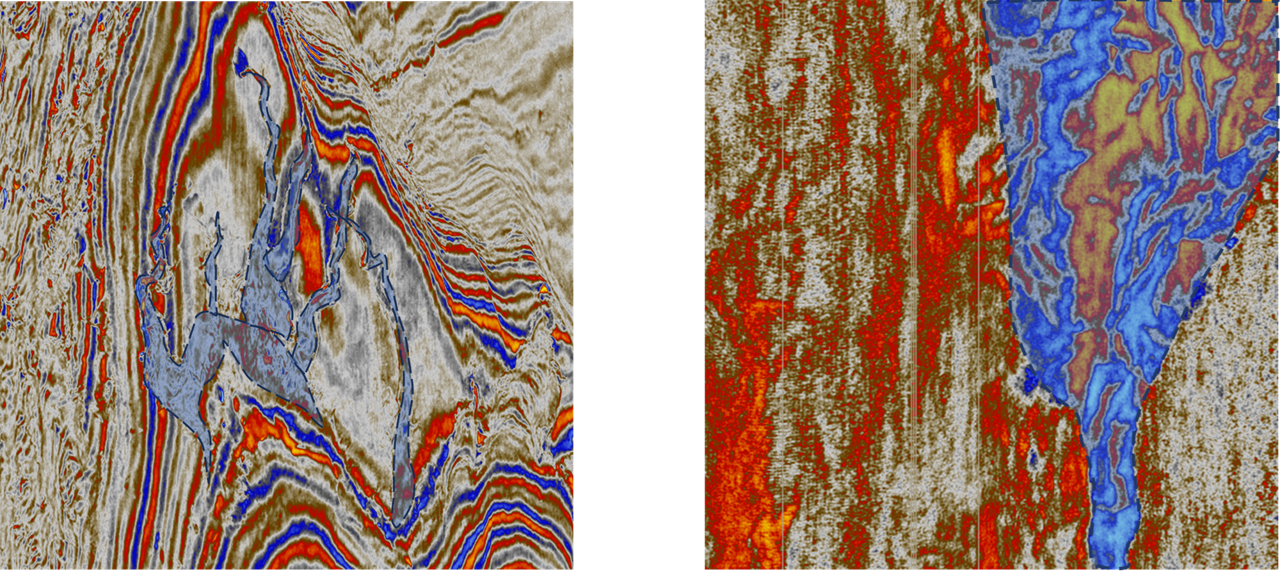
Foundation Model Approach to Automatically Discover Geofeatures
Inspired by recent computer vision breakthroughs in machine-learning (AI/ML) we are developing a seismic foundation model (FM) based approach to assist in automatically discovering geological features of interest across diverse geological environments. Whether for a single survey or hundreds of contiguous region-wide surveys, our approach permits subject matter experts to rapidly explore major geological features automatically.
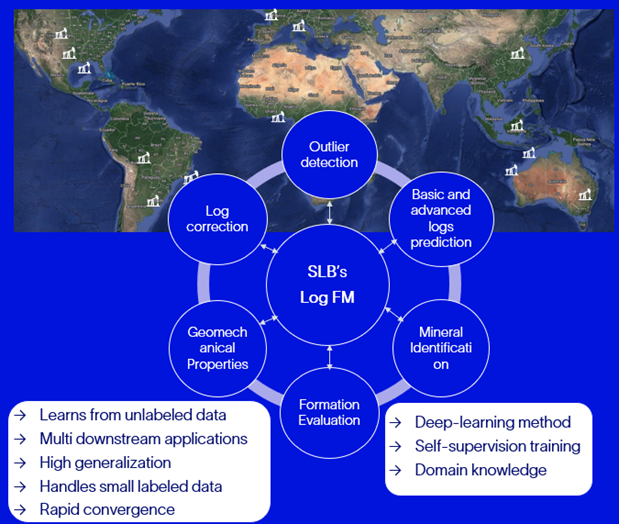
Foundational Model – A Multitask Adaptable Model for Wellbore Analysis
Envision a single neural network (NN) application that can be quickly adapted to address various log analyses, each with a fast turnaround and low computational cost. To achieve this goal, we are developing a suite to enable such capabilities and potentially reshape the future of log analysis workflow.
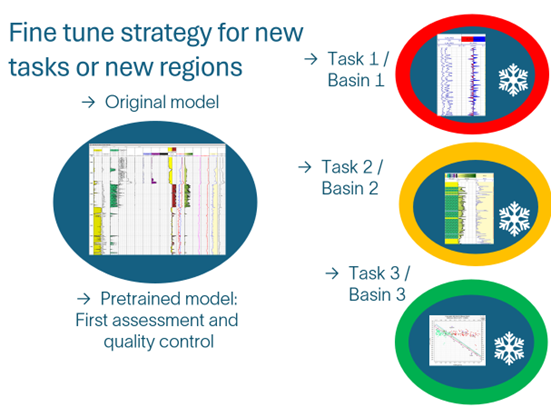
Agentic AI for log analysis – Virtual petrophysical assistant
Wellbore log analysis needs an easy-to-use framework to improve automation and consistency across multiple downstream applications, formation types and data-quality-related challenges. It also needs tools that consider the rich information from exploration wells and previous domain knowledge to provide high-quality inference in new wells containing only basic information.
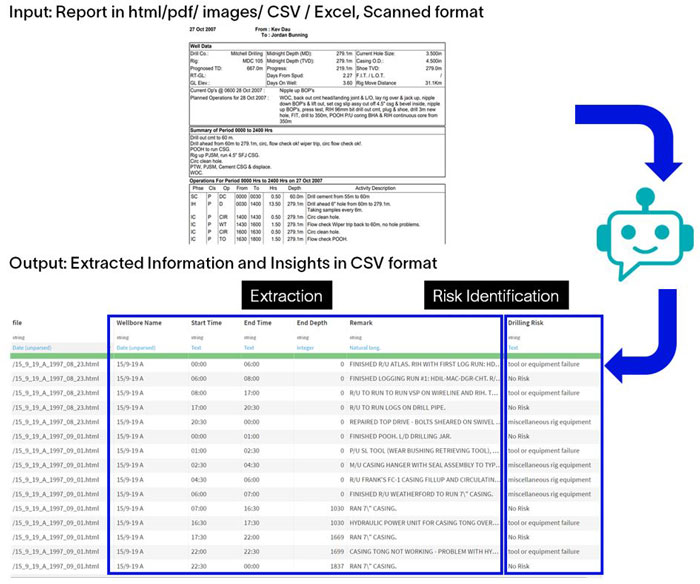
Extracting drilling risks from Daily Drilling Reports using Generative AI
Analyzing drilling risks for upcoming wells requires insights from offset well reports. Different operators commonly have different report templates, and unidentified drilling events may remain latent within the daily drilling reports(DDRs). Manual extraction from DDRs is often time-consuming, reducing risk identification quality. Natural language processing(NLP) models were developed a few years back and have been employed to automate insights retrieval. However, these NLP models require extensive labelling for supervised learning, as they may underperform for infrequently encountered drilling risks due to lack of training samples - an approach that is expensive.
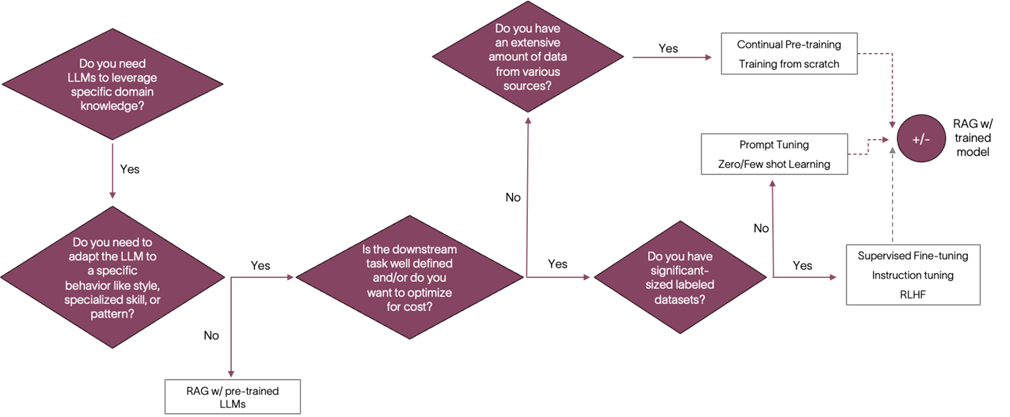
Tailoring Large Language Models for Specific Domains
Large Language Models (LLMs) excel in diverse tasks due to their broad training. However, this general-purpose nature can hinder their performance in specialized energy domains, where handling sensitive and domain-specific information is crucial. The vast technical jargon and unique language across energy disciplines pose a challenge. To unlock the full potential of LLMs in these specialized areas, targeted adaptation strategies are essential. This article explores three key approaches: prompt engineering, Retrieval-Augmented Generation (RAG), and fine-tuning. We delve into the advantages and drawbacks of each method to guide the selection for specific energy applications.
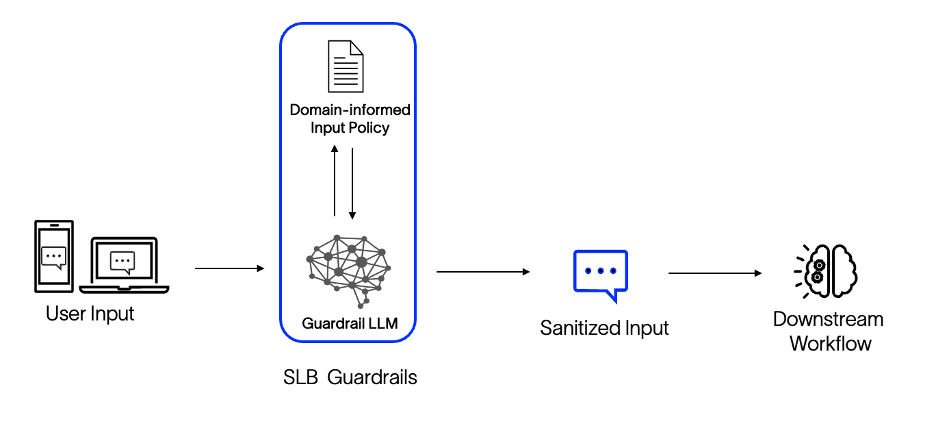
Beyond Accuracy: Building Better, Safer, and More Reliable LLM Systems
Large language models (LLMs) are revolutionizing natural language processing (NLP), offering powerful generative capabilities. While Retrieval Augmented Generation (RAG) systems hold immense promise for domain-specific applications, their reliability and safety remain critical concerns, especially in the energy industry. Unlike LLM-based chatbots built for general-purpose use, our domain workflows involve actions and decisions with significant safety and environmental implications, demanding a higher degree of reliability. The vastness of data used to train LLMs can compromise their accuracy in technical domains, highlighting the need for specialized approaches beyond simply increasing training data. This article explores two key areas for improving RAG systems: input guardrails for enhanced safety and the development of robust benchmarking methods. By addressing these challenges, we aim to pave the way for safer, more reliable, and performant LLM-based applications.
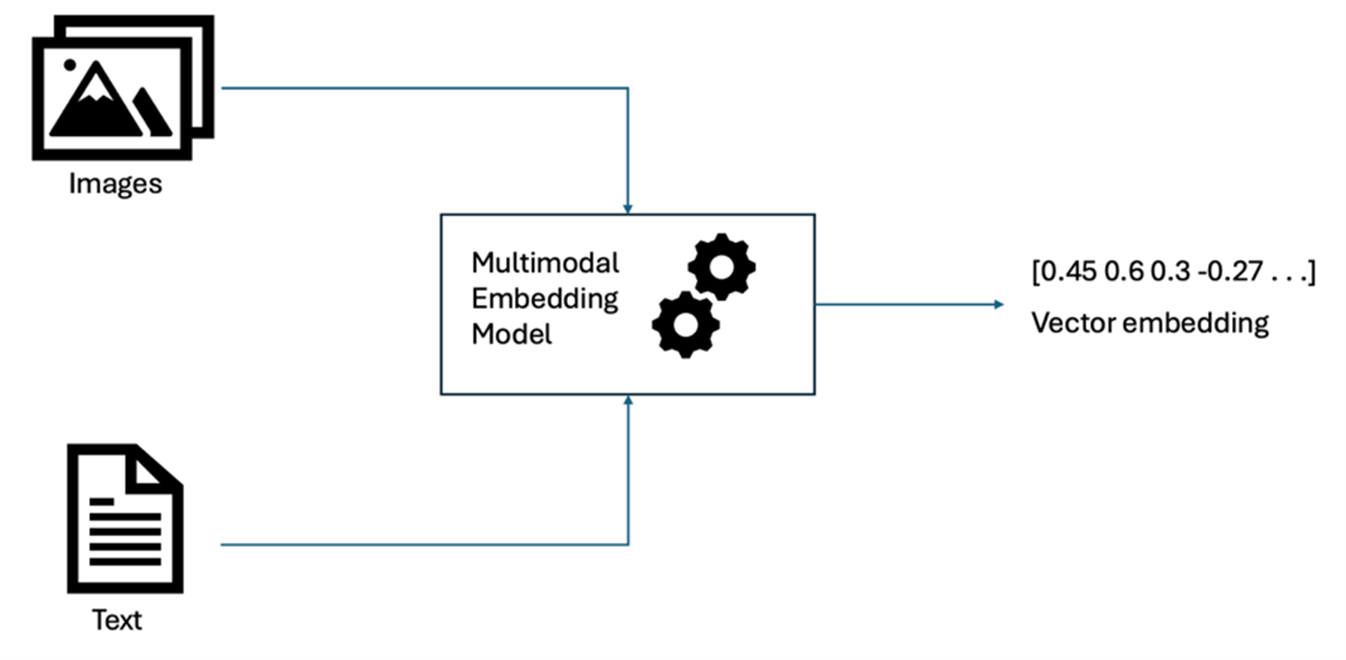
The Future of AI: Exploring Multimodal RAG Systems
The future of information retrieval is here, and it's more than just text. Multimodal Retrieval Augmented Generation (RAG) systems represent a significant leap forward, capable of understanding and responding to queries using a combination of text, images, videos, and even audio. This means we can analyze seismic data, well logs, geological maps, and even drone footage alongside text documents to provide comprehensive answers to complex energy domain questions. This article explores the power of multimodal RAG and the exciting potential it holds for revolutionizing our industry's decision-making and operational efficiency.
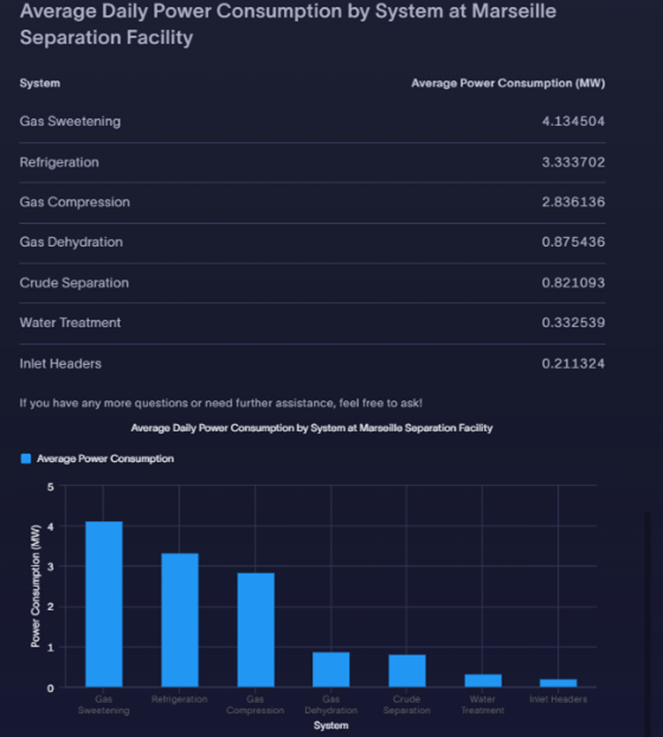
The Power of Generative AI in the Oil and Gas Industry: Enhancing Production Systems with Multi-Agent Technology
The oil and gas industry has long been characterized by its complexity, high stakes, and the necessity for precise and timely decision-making. In recent years, the advent of digital transformation has started to reshape the sector, making it more efficient, safe, and responsive to the ever-changing global energy landscape.
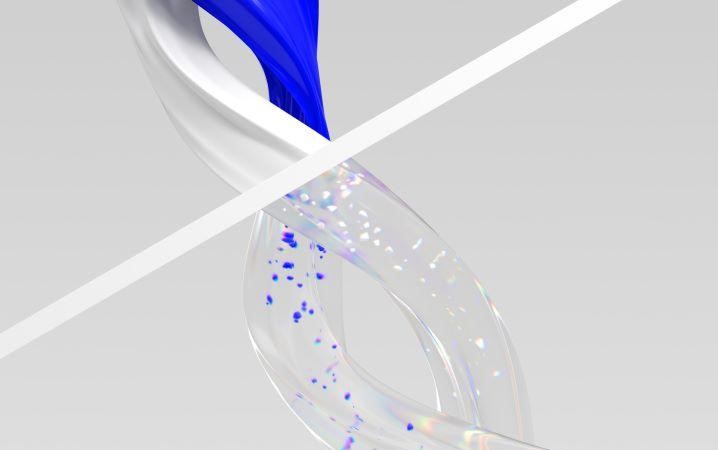
Overview of Subsurface Foundation Models
Over the past decade, machine learning (ML) has seen rapid advancements, particularly models utilizing convolutional neural networks (CNNs), generative adversarial networks (GANs), and transformers. Transformers, driven by self-attention mechanisms and self-supervised learning, have excelled in natural language processing (NLP) and have expanded into fields like computer vision (CV) and speech processing. Their success stems from their ability to capture long-range dependencies in data, scale to complex models, and leverage large datasets. These models demonstrate emergent behaviors like in-context learning, which allows them to address new tasks during inference.
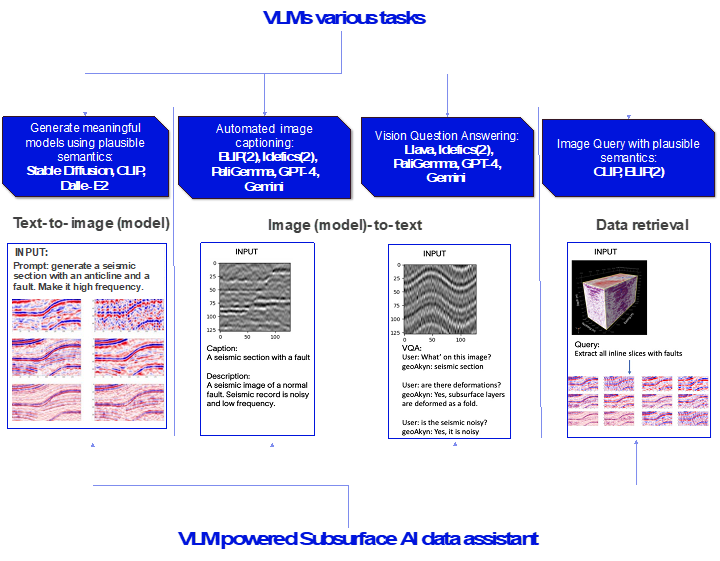
Beyond Pixels and Words: The Power of CLIP in Fusing Vision and Language
Imagine a world where geoscientists can effortlessly extract insights from complex subsurface visual data simply by asking questions in plain language. This future is rapidly approaching thanks to advancements in Vision Language Models (VLMs). By bridging the gap between the visual and textual domains, VLMs are revolutionizing industries, including the critical realm of subsurface resources.
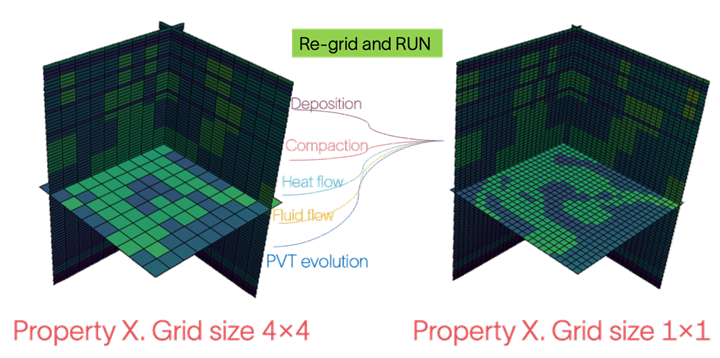
Increase Resolution of a Simulated Subsurface Property with Machine Learning in Seconds, not Hours.
Subsurface forward modeling simulation methods, such as basin modeling, can be labor-intensive. Additionally, simulation times are high at a regional basin scale. Companies face the problem of increasing the resolution of the models and reducing the simulation time. The solution we consider is a combination of basin modeling forward simulation with super-resolution machine learning architecture to predict rock properties at basin scale with higher resolution but considerably decreasing the time to simulate the properties compared to high-resolution basin modeling simulations. This solution does not inject any new information, like geostatistical methods. However, it decreases simulation time in orders of magnitude without significantly compromising the quality of the resulting property maps.
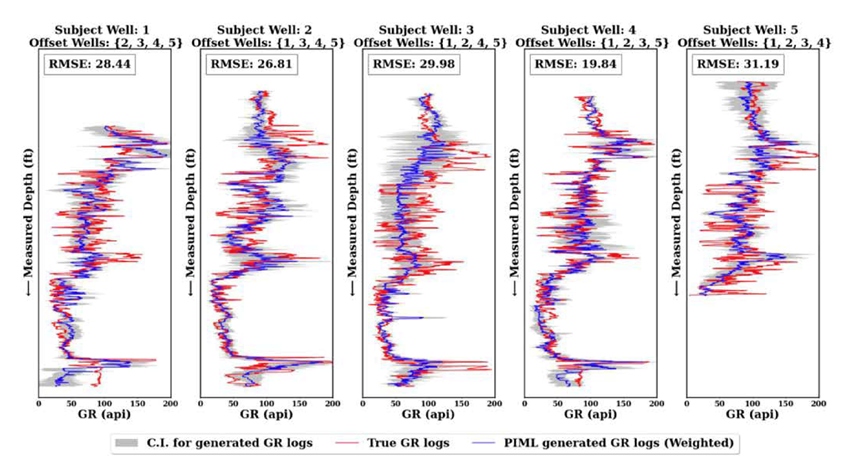
Revolutionizing well logging: Generating real-time gamma ray logs from drilling data with physics-informed machine learning
The oil and gas industry is in constant pursuit of innovation, seeking ways to optimize exploration, production, and, ultimately, profitability. A critical aspect of this optimization lies in understanding the subsurface environment, where valuable resources are locked away. Logging while drilling (LWD) has emerged as a cornerstone technology, providing real-time data about subsurface formations as drilling progresses. However, traditional LWD methods, while effective, rely on deploying sophisticated and expensive tools downhole. These tools, often susceptible to harsh downhole conditions, contribute significantly to the overall cost of drilling operations.
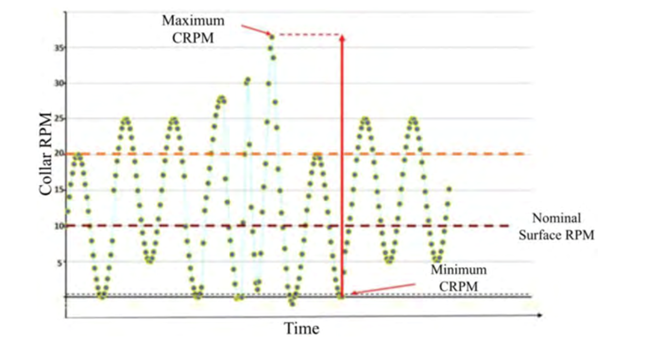
Drilling into the Future: A Hybrid Approach to Predicting Stick/Slip
Stick/slip is a common problem in drilling operations that can cause significant delays, damage to equipment, and even affect the success of the well. It happens when the energy generated by the rotary system on a drilling rig doesn't reach the drill bit efficiently, resulting in a buildup of energy in the drill string, which causes the bit rotation to speed up and slow down erratically. This can cause the drill string to stop or reverse its rotation in extreme cases.
Training a Custom Multimodal Models for Enhanced Energy RAG Solutions
Large multimodal models are a critical part of advanced artificial intelligence (AI) systems that can process and integrate text, images, audio, and video using deep-learning methods. These models excel at interpreting complex information, offering more accurate and context-aware responses. Their versatility supports a wide array of applications, from improving virtual assistants to advancing autonomous systems, pushing the limits of machine capabilities and artificial intelligence.
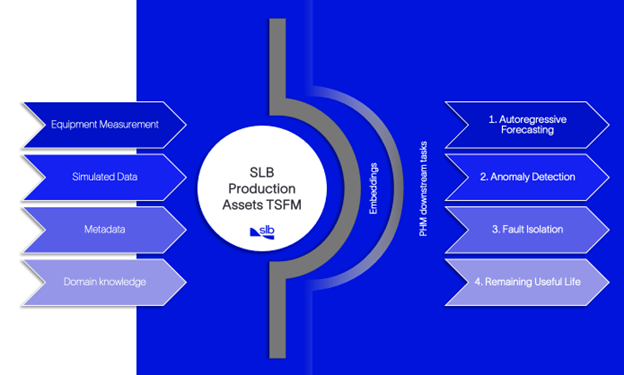
A Deep Dive into Time Series Foundation Models for SLB Surface Production Assets: Unveiling the Future
Time series data are ubiquitous across various domains and industries and holds significant importance in many real-world dynamical systems such as weather, economics, and energy of course. Due to the prevalence, techniques for analyzing time series data have been extensively studied using statistical methods, machine learning, and deep learning models. Though deep learning-based methods have been able to surpass traditional time series methods, they are still limited to one model per dataset.