Generative AI for Subsurface Workflows in Petrel
Generative AI for Subsurface Workflows in Petrel
Petrel™ subsurface software is a powerful subsurface tool designed to tackle complex problems. However, both beginner and advanced users often spend significant time not on scientific tasks, but on complex dialogs, visualizations, and displays. These tasks include adjusting labels, color tables, and configuring settings across multiple windows. While these activities are important for creating a final product, they can detract from the larger workflow at hand.
By shifting the focus away from individual process parts, we can return to the true intention of what a user is trying to achieve. Instead of fixating on intermittent steps, we can concentrate on the specific scientific outcomes a user hopes to achieve. This approach moves us away from tasks like, "I need to make polygons and then cut them out of a surface" and closer to goals like, "I want to see which of my lease blocks has the highest average porosity." Generative AI offers us an opportunity to understand and work with user intentions in a way that was previously not possible.
Workflow Intention
Users open Petrel software with specific scientific intentions in mind, and these intentions drive their series of tasks in day-to-day work. When we focus too much on the "how" of a workflow, there’s a risk of losing sight of the "why" behind it. The strength of the Petrel software AI assistant is that it reintroduces base intentions into the context of tasks a user wishes to complete. For example, a user might have the intention, "I want to QC my new seismic interpretations." The AI assistant understands this intention and translates it into a series of necessary actions to achieve this goal, remaining more flexible than code solutions like the workflow editor. With one prompt, the assistant can open multiple windows, find necessary data, and configure the proper settings, allowing the user to focus on the core scientific work without wasting time on multiple steps to get there.
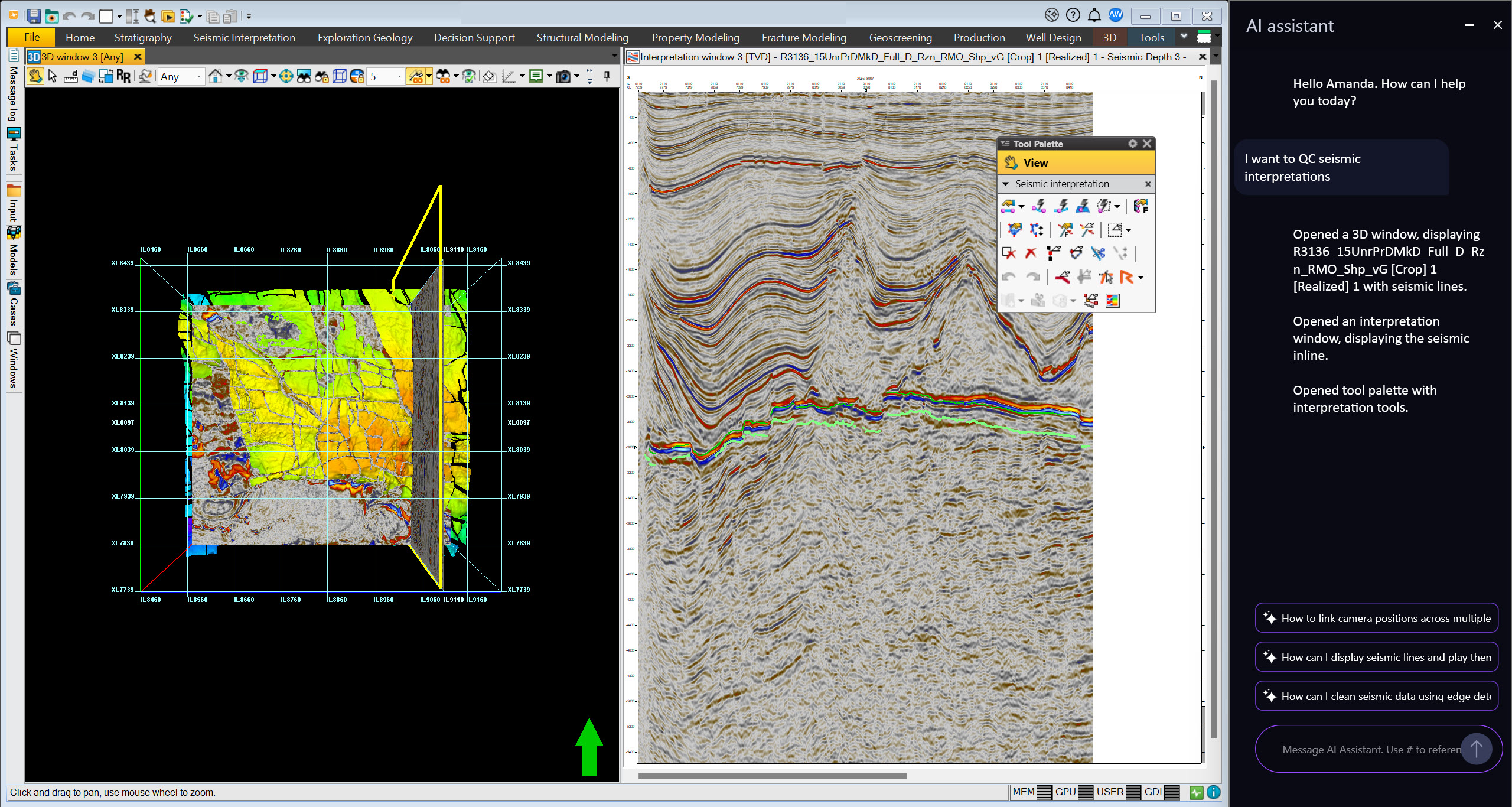
Figure 1: The assistant understands the intention for seismic QC and opens the correct windows, finds the correct data, and opens the interpretation tool palette. It then suggests other relevant actions the user could take next based on previous intention and context.
Find Data
Data is at the core of all subsurface work. New data is added to databases daily, and users continuously quality check, compare, and incorporate this new data into their workflows. A significant amount of time is often spent finding the correct data or navigating through databases, projects, and filter panels to manually query for new logs or interpretations. The AI assistant offers a streamlined way to interact with data, addressing the true needs behind multiple queries. The end goal is not to spend time with data tables but to find the needed information simply and continue with scientific work.
Users can interrogate projects with questions like, "What is my newest seismic cube and when was it imported?" The alternative would be manually checking the statistics and history of each cube, which can be tedious and time-consuming, especially in projects with multiple surveys and cubes. Finding the correct data is not the end of the workflow, but only the beginning. Traditionally, a user would manually find all wells in their area of interest with updated logs first, and then take this subset of wells into their workflow. With the AI assistant’s ability to understand context and intention, a user can combine this data criteria into their true intentional prompt, such as, "Make a model with my newest surfaces and porosity logs." In this way, the AI assistant fundamentally reimagines the experience of finding data within the context and intention of the user's work.
Complex Support Queries
Manuals and support documentation are extremely helpful; however, it can be nearly impossible to write documentation that fully fits a user’s unique workflow. Users around the world have the power to tailor workflows and processes to their specific needs, but it is challenging for a single piece of documentation to meet the needs of thousands of people. The AI assistant can leverage its understanding to navigate and merge articles, better addressing the user’s intent and needs. Instead of parsing multiple support articles, the assistant can deliver a customized result that fits the user's requirements, providing better assistance more quickly.
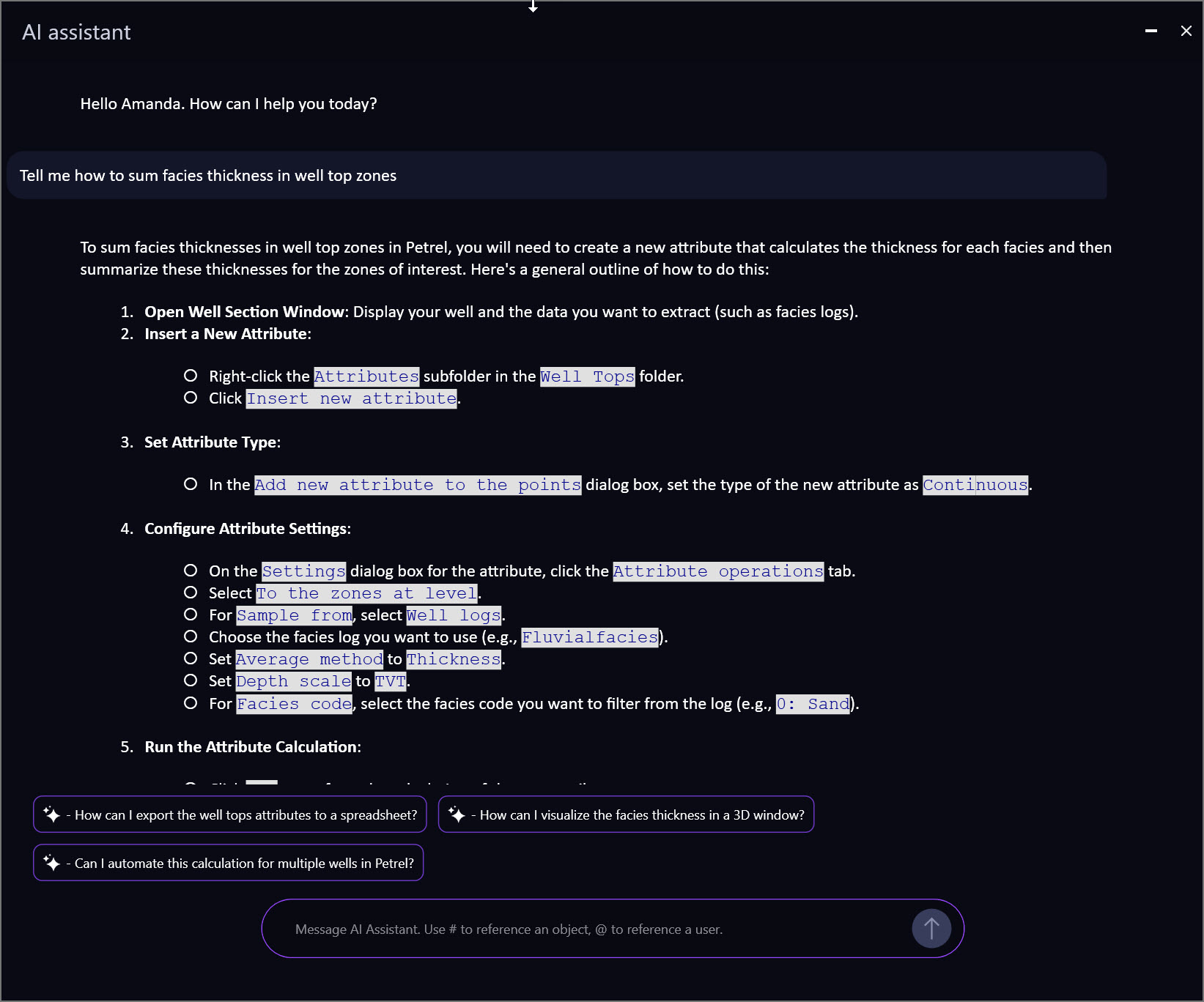
Figure 2: Example of a question that requires a combination of multiple help articles to answer. The AI synthesizes this into one succinct flow for the user.