Pretrained Models for Rapid Geofeature Segmentation
Pretrained Models for Rapid Geofeature Segmentation
Transformer-based architectures can scale complex models and meaningfully process large volumes of seismic data. Architectural constructs within these models can allow multi-scale capture of seismic data. Self-supervised learning methods allow these models to be trained on very large datasets without needing labeled data. Models built in such a fashion, so-called “pretrained models”, can exhibit feature representations which are more complete, provide better generalization, and implicitly capture diverse domain-specific semantic nuances. Thus, features from such pretrained models can be repeatedly used as a starting point for various downstream applications by quickly repurposing them, using very few labels. Figures 1-3 provide examples of employing such an approach to rapidly segment foresets, channels, and shallow gas.
![]() | ![]() |
Figure 1a. Foreset segmentation across Parihaka. The segmentation model is rapidly built using the pretrained seismic FM and very few labels. This lightweight trained model is used to segment the foresets across the survey. The multi-class foresets are overlayed with red, yellow and blue colors. Figure 1b provides a sectional view.
To tackle numerous seismic interpretation problems, we have employed transformers to build seismic foundation models (FM). We have employed these pretrained models to build a system for geofeature discovery. When applied to unseen seismic surveys, the system can discover a broad set of geological features (see the accompanying blog: Geofeature Identification). We believe our pretrained seismic models capture multi-scaled local and contextual semantic nuances of seismic data resulting in the ability to easily accomplish tasks on seismic data the model has never seen before. One may view a large-scale pretrained seismic FM as a means to nonlinearly project raw seismic data onto a higher-dimensional semantically structured feature space. In such a feature space, complex interpretation goals are more robustly accomplished using smaller models (SM) and far fewer labels than traditional machine-learning methods. For example, using features from the pretrained seismic FM, and providing a few samples of the segmentation labels for training, the expert can rapidly train an SM to conduct multi-class semantic segmentation of geobodies (see Figures 1-3). Labels (image-like annotations, not short captions) are one means of conveying the interpretation intent. Labels may be directly employed for training purposes (above example), or under other learning paradigms, labels may be employed in a few-shot manner. Labels convey the user intent and are the equivalent of user query/prompts in large language models (LLM). Thus, a few labels are required to clearly convey the intent to the model. The prime reason for this elaboration is to suggest that, within reason: (a) multiple interpretation tasks can be simultaneously accomplished when expressed together in a single label or across labels; and (b) expressing intent via labels provides the expert with an opportunity to creatively rework/consolidate parts of the traditional seismic interpretation workflows. Such multi-tasked formulations may shorten interpretation workflows. To allow for such use, we adopt labeling to accomplish various interpretation tasks. SLB is currently working towards integrating such seismic FMs in Delfi to further strengthen the next generation subsurface interpretation workflows.
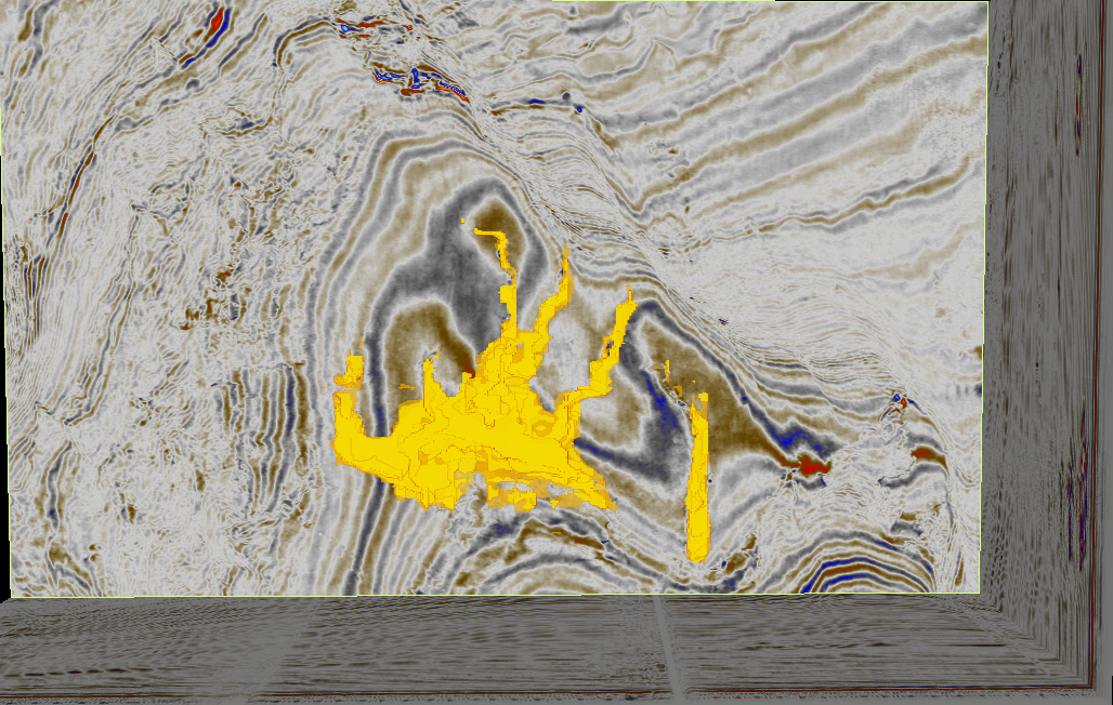
Figure 2. Channel segmentation within Parihaka. Using a few labels, and our pretrained seismic FM, the expert can rapidly build a model for such geobody segmentations.
Continuing with segmentation as an example interpretation task, we use the features from our pretrained seismic FM as input to a small model (SM) and train it using very few multi-class segmentation labels. Figures 1-3 show examples wherein this approach has been used to segment foresets, channels and shallow gas. Figures 1a, b shows the performance of this approach for multi-class segmentation of foresets on Parihaka 3D seismic data. While we show a few performance samples here, this tuned machine has segmented the entire survey remarkably well (Figure 1b)!
![]() | ![]() |
Figure 1b. A sectional view of the foreset segmentation results for Parihaka. The multi-class foresets are overlayed with red, yellow and blue colors. See Figure 1a for the segmentation performance when the model is applied to the entire survey.
Acknowledgements: We would like to thank the New Zealand Petroleum and Minerals (NZPM), Ministry of Business, Innovation & Employment, New Zealand, for providing the access to data used in our study.
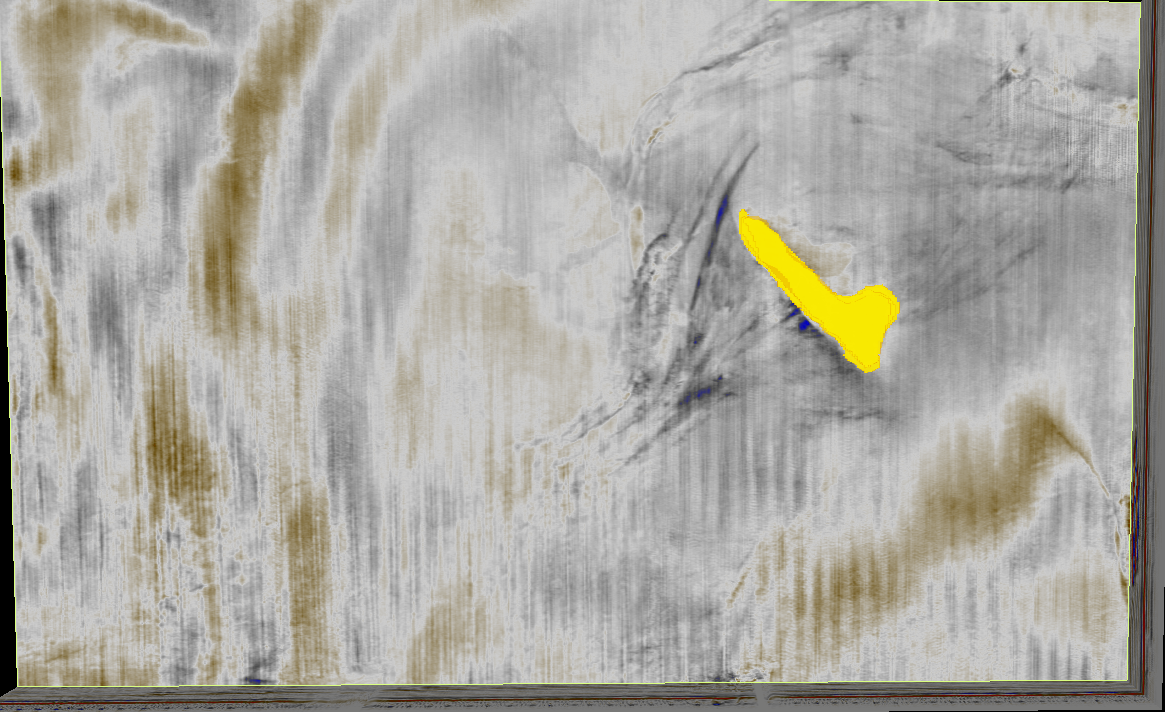
Figure 3. Shallow gas segmentation within Parihaka using our pretrained seismic model approach.