Driving Innovation in Subsurface Simulation Workflow Using Generative Artificial Intelligence
Overview of the Smart Production Assistant
Recently, generative artificial intelligence (GenAI) has taken every industry by storm. For those who are new to this term, here is a simple definition as generated by Microsoft® Copilot®:
Generative AI refers to artificial intelligence systems that can create new content, such as text, images, videos, or audio, based on the data they were trained on. Unlike traditional AI, which focuses on analyzing existing data to make predictions or decisions, generative AI uses neural networks to generate novel outputs that mimic the style and structure of the data it was trained on. This capability allows generative AI to produce creative works, making it distinct from other AI applications that are typically more analytical or predictive in nature.
In this blog, we will focus on a suite of applications that enable the use of GenAI in simulation workflows. These applications are aimed at improving efficiency and enabling workflows that were not possible or very time consuming using traditional non-GenAI driven approaches. AI is already making big strides in reservoir simulation workflow by enhancing accuracy and efficiency of predicting the response from the subsurface and optimizing strategies to enable decision making. Some of the key applications are:
- Predictive modeling: Machine learning (ML) methods are now used extensively to predict the dynamic response from subsurface models under varying controls at the surface. This allows reservoir and production engineers to forecast reservoir performance and extract maximum value.
- History matching: AI-assisted history matching has been presented in recent literature and is quickly gaining popularity. Along with improving the performance of these workflows, AI is enabling integration of highly disparate physical scales.
- Real-time monitoring and control: AI enables real-time monitoring and control of reservoir operations. Data collected from edge devices provides valuable insights on field operations.
Traditional ML/AI methods are finding applications in improving the efficiency of very complicated workflows but there are several needs that are unmet. Let’s take an example of the uncertainty quantification workflow. The following steps are used in an iterative workflow that enables reservoir and production engineers to make informed decisions towards field operations:
- Data collection and preparation
- Identify key variables
- Define uncertainty ranges
- Generate scenarios
- Run simulations
- Analyse results
- Sensitivity analysis
- Visualization
- Decision making
GenAI can now assist with every step from data collection to aiding decision making.
Application to ensemble workflows
The figure below shows how we are enabling automatic generation of multiple subsurface models, even for the most complex reservoirs, delivering rapid insights to support building Field Development plan recommendations in an operational timeframe.
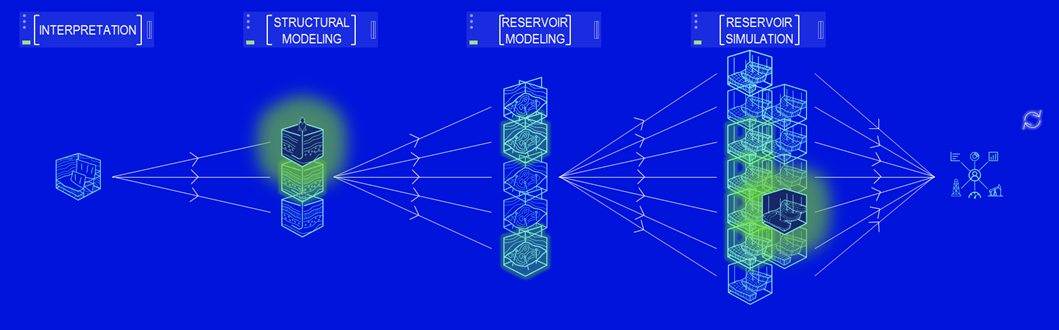
GenAI is now enhancing similar workflows by providing advanced processes such as:
- Ability to collect data from structured and unstructured data sources, thereby reducing the manual processing tasks and improving efficiency.
- Create simulation components such as fluid models, rock physics, field management strategies, grid properties, etc. from minimal user input and automated object generation.
- Generate a range of realizations with varying properties using simple user prompts.
- Enable orchestration and running of ensembles using SLB APIs.
- Real-time and customized results and insights generation from the ensemble data.
- Customized and on-demand visualization of reservoir dynamic and static properties including well reports.
- Assisted decision making and reports generation.
Example: uncertainty quantification workflow
Uncertainty quantification workflow involves a series of complex steps each requiring a set of expert knowledge and software. Below are some of the steps that show the complexity of executing such workflow. First the various components such as fluid models and rock models need to be constructed. These are normally constructed using software such as fluid modelers and processing lab data to simulation model inputs. Each of these steps can now be performed using simple natural language queries.
- Generate surface conforming grids, compositional fluid models, initial conditions, wells and completions, and field management strategy.
- Generate Intersect™ high-resolution reservoir simulator base case (Gomes et al. 2022) using the above information and components.
- Generate rock models using uncertain parameters.
- Generate an ensemble of models using the various rock models generated in the above step.
- Orchestrate and run the ensemble of simulations using the Delfi™ digital platform on-demand reservoir simulation (https://www.slb.com/products-and-services/delivering-digital-at-scale/software/delfi/delfi-solutions/delfi-on-demand-reservoir-simulation)
Combing GenAI within our workflows allow us to execute all the steps listed above in one go.
The highlight: this entire workflow can be orchestrated and run with one simple prompt!
Using the base case, run an uncertainty quantification workflow using 50 realizations by varying gas Corey parameters between 3.6-7.6.
The Future of AI in subsurface workflows
The integration of Generative AI into subsurface workflows will bring transformative changes, enhancing efficiency, accuracy, and innovation in our operations. We invite other energy companies to collaborate with us in leveraging these cutting-edge technologies to unlock new possibilities and drive the future of energy together.
Gomes, C. J., Geiger, S., and Arnold, D.: “The Design of an Open-Source Carbonate Reservoir Model,” Petroleum Geoscience, (2022) 31 August. https://doi.org/10.1144/petgeo2021-067.