Trusting GenAI: Crafting a Robust Platform Strategy for the Energy Sector
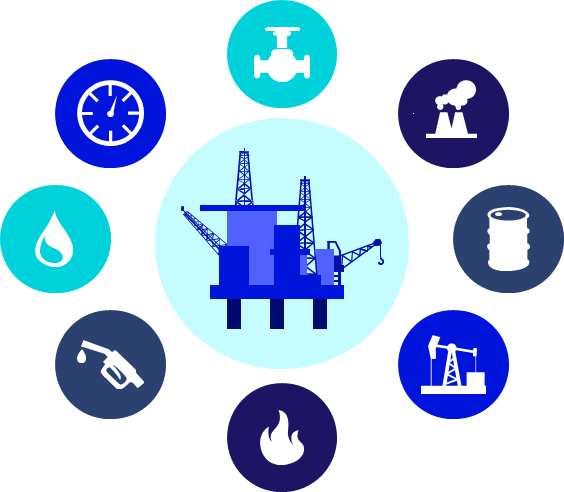
Introduction
We stand on the brink of a profound transformation. Artificial intelligence is rapidly reshaping the entire value chain within the energy sector, with the emergence of Generative AI (GenAI) compressing years of evolution into mere months. However, a critical challenge persists: fostering trust in AI-driven decisions, especially in an industry where the stakes are exceptionally high.
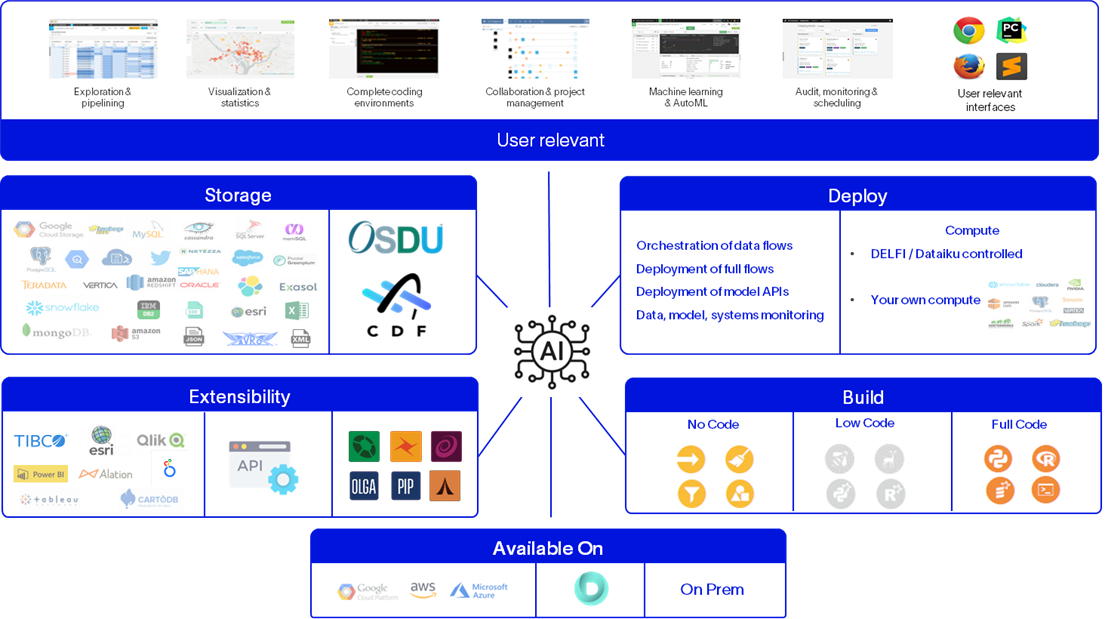
The High Stakes of AI in Energy
In the energy sector, the "cost of failure" in field operations can be catastrophic, potentially leading to loss of life and environmental damage. Consider this stark contrast:
- In retail, if an ML model incorrectly suggests buying a TV when purchasing a phone, the consequences are minimal.
- In energy, an incorrect prediction of mud losses during drilling could result in a disastrous oil spill.
This disparity underscores the crucial need to build and maintain trust while integrating new technologies in the energy sector.
Key Factors for Successful AI Adoption in Energy
To achieve this delicate balance of innovation and trust, we must focus on three critical factors: Democratization, Scalability, and Agility.
- Democratization: Bringing Everyone to the Table
Democratization in AI platforms means creating an environment where various stakeholders in the value chain can collaborate effectively. This approach is vital for several reasons:
- Enhanced Trust:By involving all responsible parties in developing data-driven workflows, we build confidence in the decision-making process.
- Unified Platform: Imagine a tool where everyone, regardless of their role, can interact with the value chain, contribute their expertise, and understand the implications of AI-driven decisions.
- Breaking Down Silos:While some energy firms have adopted integrated approaches like reservoir studies, true democratization goes beyond user collaboration to system-facilitated interaction.
Example: An offshore drilling operation uses SLB’s democratized AI platform. The geologist's rock analysis, the drilling engineer's equipment specifications, and the environmental scientist's ecological impact assessment all feed into the same system. This holistic approach leads to more informed, trusted decisions about drilling locations and methods.
- Scalability: Handling Data from Megabytes to Terabytes
In the energy sector, data volumes can range from a few megabytes to terabytes. A robust AI platform must be able to scale accordingly:
- Flexible Infrastructure: The system should handle varying data loads without burdening domain experts with technical concerns.
- Cost-Effective Scaling: While scalability is crucial, it must be balanced with cost considerations to avoid runaway expenses.
- Seamless User Experience:Whether working with small datasets or massive information banks, the user interface and functionality should remain consistent and efficient.
Example: SLB's AI platform seamlessly scales from analyzing daily production data from a single well (megabytes) to processing seismic data for an entire oil field (terabytes). This scalability allows for consistent decision-making processes across different project scales.
- Agility: Adapting to Rapid Technological Advancements
The pace of AI advancement, particularly since the advent of large language models, necessitates an agile approach:
- Quick Integration: Platforms must be designed to swiftly incorporate emerging technologies.
- Flexible Workflows:: As new AI capabilities emerge, workflows should be easily adaptable without requiring complete overhauls.
- Continuous Learning:Both the platform and its users should be in a state of continuous learning and adaptation.
Example: When GPT-3 was released, an agile SLB AI platform quickly integrated its natural language processing capabilities. This allowed engineers to interact with complex drilling data using conversational language, dramatically reducing the time needed for data analysis and decision-making.
SLB's Generative AI Platform: A Case Study in Energy-Focused AI
At SLB, we've developed a state-of-the-art generative AI platform tailored specifically for the energy sector's unique demands. This cutting-edge solution embodies the principles of democratization, scalability, and agility:
- It allows diverse teams to collaborate on a single platform, from geologists to environmental scientists.
- The platform scales effortlessly from individual well analysis to entire field operations.
- Its modular design allows for quick integration of new AI technologies as they emerge.
Conclusion: The Future of AI in Energy
As we navigate the complex intersection of AI and energy, trust remains paramount. By focusing on democratization, scalability, and agility, we can create AI platforms that not only drive innovation but also instill confidence in their users and stakeholders. The energy sector's adoption of AI is not just about technological advancement; it's about creating a safer, more efficient, and more sustainable future for energy production and consumption.
Call to Action
Are you ready to explore how AI can transform your energy operations while maintaining the highest standards of safety and reliability? Contact SLB today to learn more about our generative AI platform and how it can be tailored to your specific needs in the energy sector.