Agentic AI for log analysis – Virtual petrophysical assistant
Agentic AI for log analysis – Virtual petrophysical assistant
Wellbore log analysis needs an easy-to-use framework to improve automation and consistency across multiple downstream applications, formation types and data-quality-related challenges. It also needs tools that consider the rich information from exploration wells and previous domain knowledge to provide high-quality inference in new wells containing only basic information.
With this goal in mind, our team is developing a foundation model capable of providing direct inferences for multiple common tasks that can be adaptable to multiple applications and formation types, to be used as a core piece in a framework to help geoscientists achieve higher-quality, consistent interpretation considering multi-well information.
We envision using the latest generative technologies to enable automation in our end-to-end wellbore analysis, and we see good prospects in using agentic AI workflows to enable the machine to take decisions to improve the selection of work steps, automate iterations for data selection, propagate answers for the entire field evaluation, estimate uncertainties and perform results assessment until a successful interpretation is achieved.
We focus on user privacy and control, by offering a proprietary model version that learns from new user interactions and examples, with the capability of adapting for best practices and new data along the interpretation as indicated in Figure 1. This dynamic model learns from expert knowledge for processing and interpreting wellbore logs and enables teams to extrapolate this knowledge when interpreting new wells and fields without sharing the information outside your company.
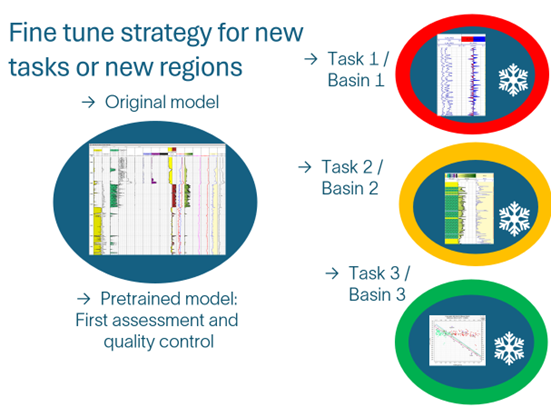
Figure 1: Strategy to give users privacy and control: We use a pretrained foundation model and adapt it to different tasks and regions creating private adapted models that learned from local aspects and expert knowledge via examples and provide answers compatible with the user best practices for well log analysis.
So far, one piece of the framework is the foundation model that is pretrained and validated in multiple wells in various datasets. This model is capable of handling inputs with different degrees of quality issues and can be adapted to multiple downstream tasks that have been developed and tested using multiple datasets as indicated in Figure 2. When building this foundation model, we focus on quality, stability, and computational efficiency, as well as improving usability. We are building customizable workflows to provide end-to-end applications including log quality control, formation evaluation, elastic properties prediction, reflection coefficient estimation, mineralogy estimation, and general log interpretation to transform our framework into a smart petrophysical assistant able to consider a large amount of data into the interpretation.
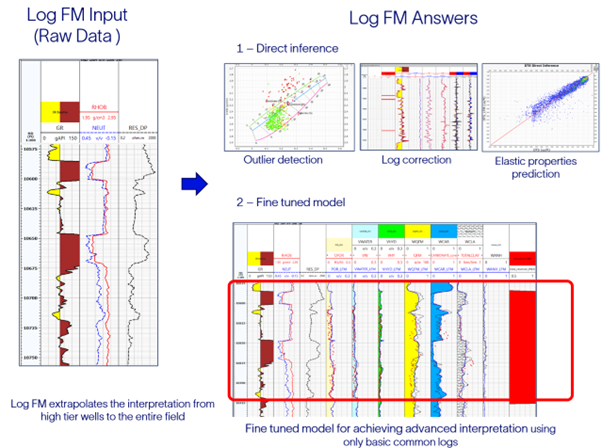
Figure 2: Examples of downstream applications enabled by large models to provide quality control and formation evaluation answers and to be executed in the background by the petrophysical assistant.
Multiple steps in the proposed workflow rely on large deep learning models that are shaped to be easily orchestrated by agents capable of selecting and executing subtasks. These agents will execute and optimize complex workflows dealing with different data-quality issues and end applications as indicated in Figure 3. One good prospect is having a data expert agent capable of selecting high quality intervals and dividing them into train, validation and test set to be used by the refined interpreter agent that adapts the Log Foundation Model to the relevant downstream tasks, the results from the interpreters can be evaluated by the reviewer agent that can access the model performance and compare with additional available data.
We pay attention to performance evaluation and uncertainty analysis, as well as the impact of multiple decisions on the final interpretation. This permits us to value transparency and improve the workflow quality and user trust along the development process. One of our goals is to increase the assistant capabilities to include the knowledge of geologists and upgrade it to a cross-discipline geoscientist assistant.
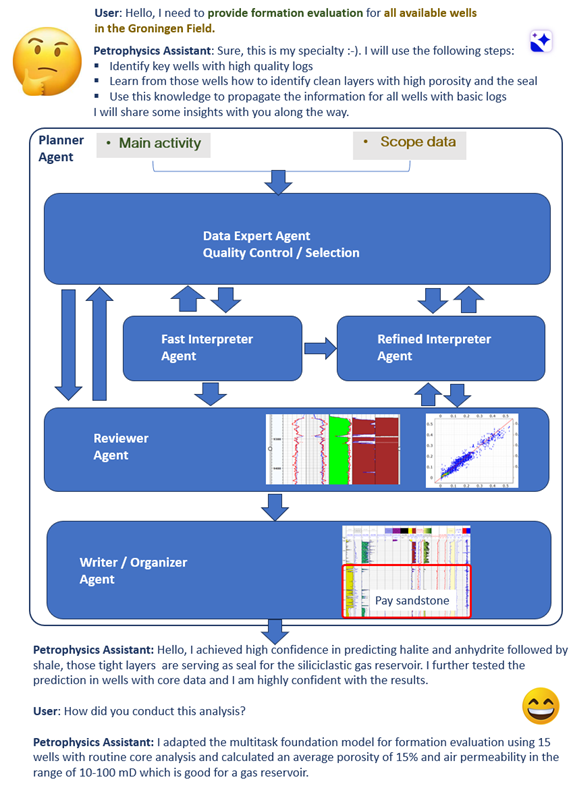
Figure 3: Example of envisioned workflow using multiple AI agents for performing wellbore log interpretation that is activated when the user starts our petrophysical assistant. Multiple activities in the background provides the user with high-quality interpretation associated with the user-defined main activity and scope data. Some examples of the main activities can be: defining zones with potential for producing hydrocarbons, zones with risks of break-out, or zones with swelling shales. Examples of scope data might be measured and interpreted logs and cores from multiple wells in a field.